Understanding the intricacies of user identity is key to successfully navigating today’s evolving digital marketing landscape. Identity graphs (ID graphs) can be used to connect the complex web of data points, cookies, and user profiles and establish a singular, unified view of a consumer.
In our previous post, we explored what an ID graph is. Below we take a look at how they work their magic behind the scenes.
Step 1: Data Collection
To be able to connect fragmented data points, it’s important to first collect various types of identifiers from multiple sources – device IDs, browser cookies, universal ID, purchase history, and personally identifiable information – both online and offline.
Step 2: Data Integration
The collected data is then ingested into a centralized database. The goal is not merely to gather the data, but to prepare it for the next steps. Here, data is cleaned from any irrelevant or redundant entries. Next, data is standardized into a common format that can be easily processed and analyzed.
Step 3: Identity Resolution
One of the key technologies that make ID graphs so powerful is the way they link various identifiers to construct a cohesive customer profile. Known as identity resolution, this is the core phase that is generally achieved using deterministic and probabilistic matching methods.
Deterministic matching: links identifiers that are definitively associated with a single user. This method uses known customer information, such as hashed email addresses or logged-in data.
Probabilistic matching: uses machine learning and statistical models to make likely connections between identifiers and users. This method is mostly used for anonymized identifiers, such as browser type, IP address, location, operating system, etc.
ID graphs may use either one of these methods or a combination of both for identity resolution.
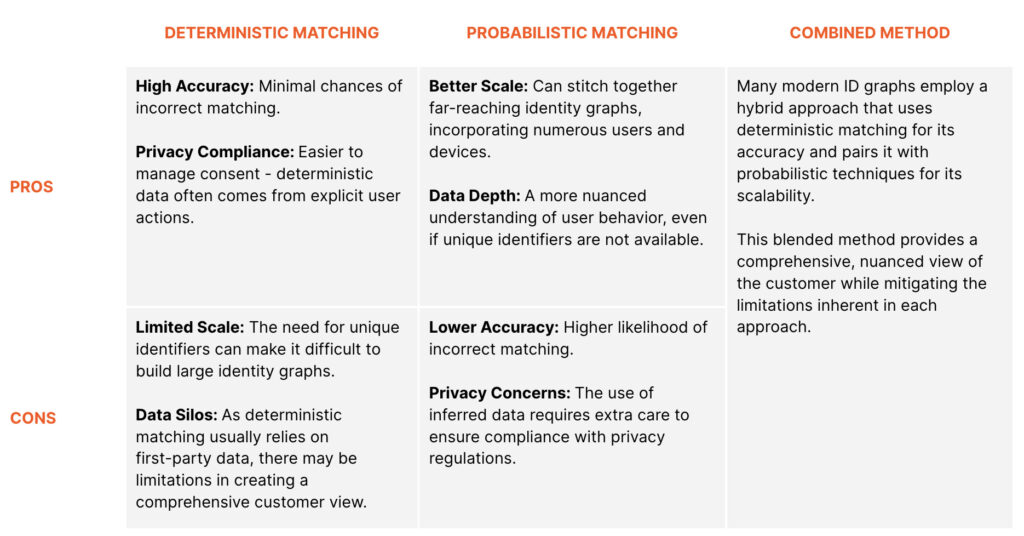
Step 4: Identity Enrichment
The ID graph can be enriched by integrating third-party data or other supplementary information to create more complete customer profiles.
Step 5: Activation
Once the ID graph is fully constructed and enriched, it is then made actionable for various use cases in digital advertising:
Audience segmentation: classify the unified customer profiles into different segments based on behavior, demographics, etc.
Targeting: use the segments to deliver personalized advertisements.
Measurement and attribution: analyze how different channels and touchpoints contribute to conversions and ROI.
Step 6: Ongoing Maintenance
People buy new devices, change jobs, and move cities throughout the course of their lives. Therefore, an ID graph is not a one-off project, but a dynamic entity that needs ongoing updates and management. ID graphs need to be regularly refreshed with new data and validated to maintain quality.
While ID graphs are complex technologies that require significant time and financial investment to set up and operate, the benefits they offer make them a crucial tool for success in navigating the cookieless future.
In part III of this series, we’ll explore the use cases and benefits ID graphs offer advertisers and publishers and how you can get started.
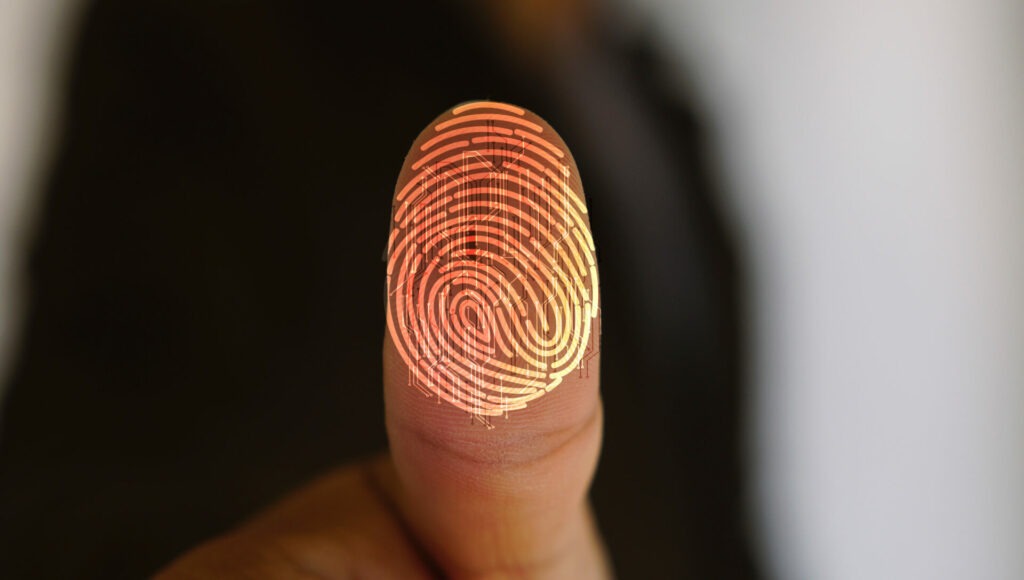
Want to learn more?