A New Approach to Data Management & Collaboration
In today’s data-driven advertising landscape, the competition for consumer attention is intense. Advertisers, agencies, and brands are constantly searching for more effective ways to target their audience, while publishers aim to monetize their inventory more efficiently. In an era of increased privacy regulations, industry players need to adapt and transform their digital marketing practices to strike a balance between personalized advertising and data privacy.
Data Clean Rooms (DCRs) are set to change the way we handle data without compromising security, and advertisers and publishers alike should consider adding them to their arsenal of addressable solutions.
What Are Data Clean Rooms?
A Data Clean Room is a secure, privacy-safe environment where first-party data from multiple parties can be combined and analyzed without leaving its source. Imagine it as a “neutral zone” where data sets from advertisers, agencies, and publishers can mingle, but personal identifiers are anonymized and safe due to strict security and governance to protect user privacy and prevent data leakage. This way, brands can leverage data from multiple sources to derive actionable insights without violating data protection regulations like GDPR and CCPA.
As a quick reminder – first-party data are pieces of information about customers and prospects collected and owned by the company across their online and offline sources. Such data is either collected from websites and apps with technical anonymous identifiers or provided by users directly when interacting with the company’s touchpoints – like signing up for a newsletter. First-party data shared with the brand – such as name, mailing address, email address, and phone number – is also known as personally identifiable information (PII).
All such types of identifiers can be fed into the DCR and matched against each other to provide additional audience and CRM data insights to facilitate more efficient, scalable, and data-driven advertising and media monetization. Matching sometimes requires a common identifier, and that’s where identity graphs (ID graphs) come into play.
As ID graphs can identify two bits of information belonging to the same user to form a consistent, unified view of the customer, clean rooms can rely on ID graphs to match and reconcile various types of identifiers.
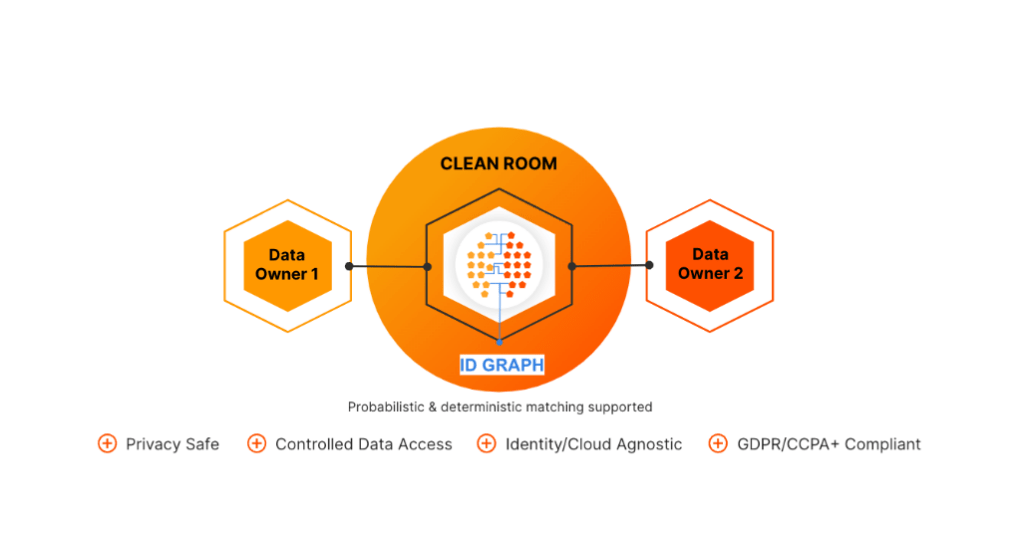
Data Clean Room Applications
Data clean rooms are powerful and versatile, but they must be operated with expertise and structure to deliver relevant outcomes. While some of the many DCR applications can be very specific to an advertiser’s or publisher’s needs, they can also be used for:
First-party targeting
Example: An advertiser wants to target their CRM user base with a targeting campaign across the web without sharing any data with publishers.
Solution: Match advertiser first-party data against Equativ publisher first-party data and target the matched data set.
Closed loop performance measurement
Example: An advertiser wants to measure the conversion of their online campaign based on offline purchase behavior through their customer’s loyalty card purchase behavior.
Solution: Onboard the reporting data from the online campaign and match it against the first-party data from the CRM of the loyalty cards.
Extend addressable audiences
Example: An advertiser is using a DMP to target their first-party data. This DMP is pushing cookies to Equativ. The ID graph will allow this advertiser to target or retarget their first-party data on other environments (in-app, CTV, etc.).
Solution: Match cookies against MAIDs or CTV IDs.
Target households based on user data
Example: An advertiser wants to target clients in their homes on their CTV devices. They match personal data, such as mobile numbers, with household IDs to do so.
Solution: Match user IDs against household (HH) IDs.
Cross-device measurement
Example: An advertiser serves a video ad to a user on their desktop. Then, the user points makes use of an app on their mobile device to convert. The ID graph will allow attributing the conversion to the original device/campaign.
Solution: The ID graph will help with the conversion, reconciliation, and deduplication.
Ultimately, DCRs can help make smarter data-driven decisions based on attributed data touch points to reach target audiences at scale, attribute and measure ad performance in cross-device and cross-channel campaigns, and identify untapped revenue opportunities based on a deeper understanding of user behavior.
In part II of this series we break down the benefits and limitations of the technology and explain how to get started.
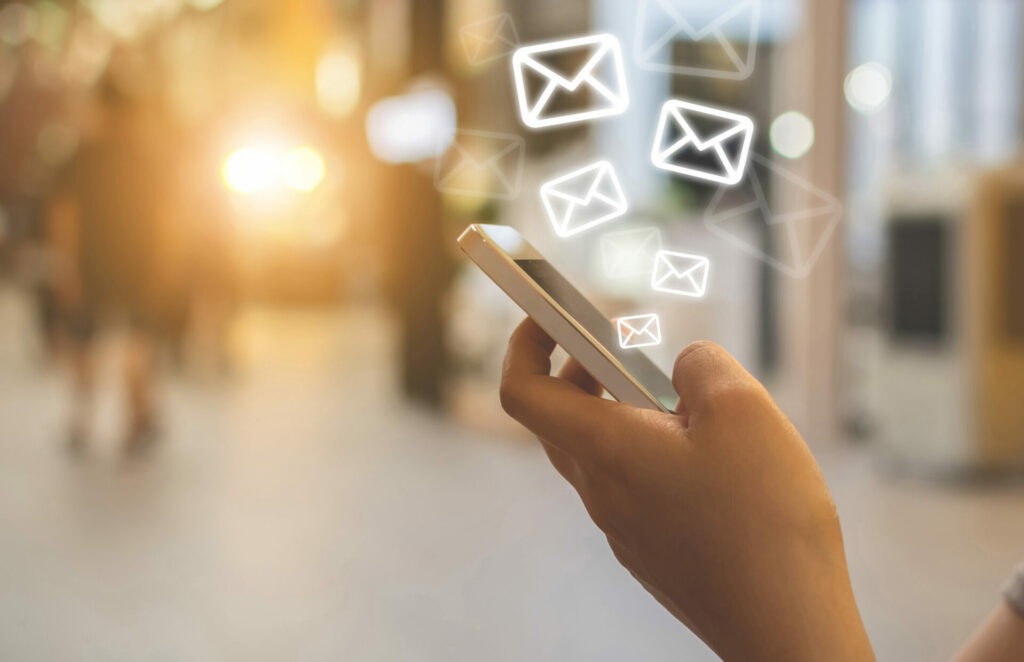
Want to learn more?